The Revolution of Machine Learning in Healthcare
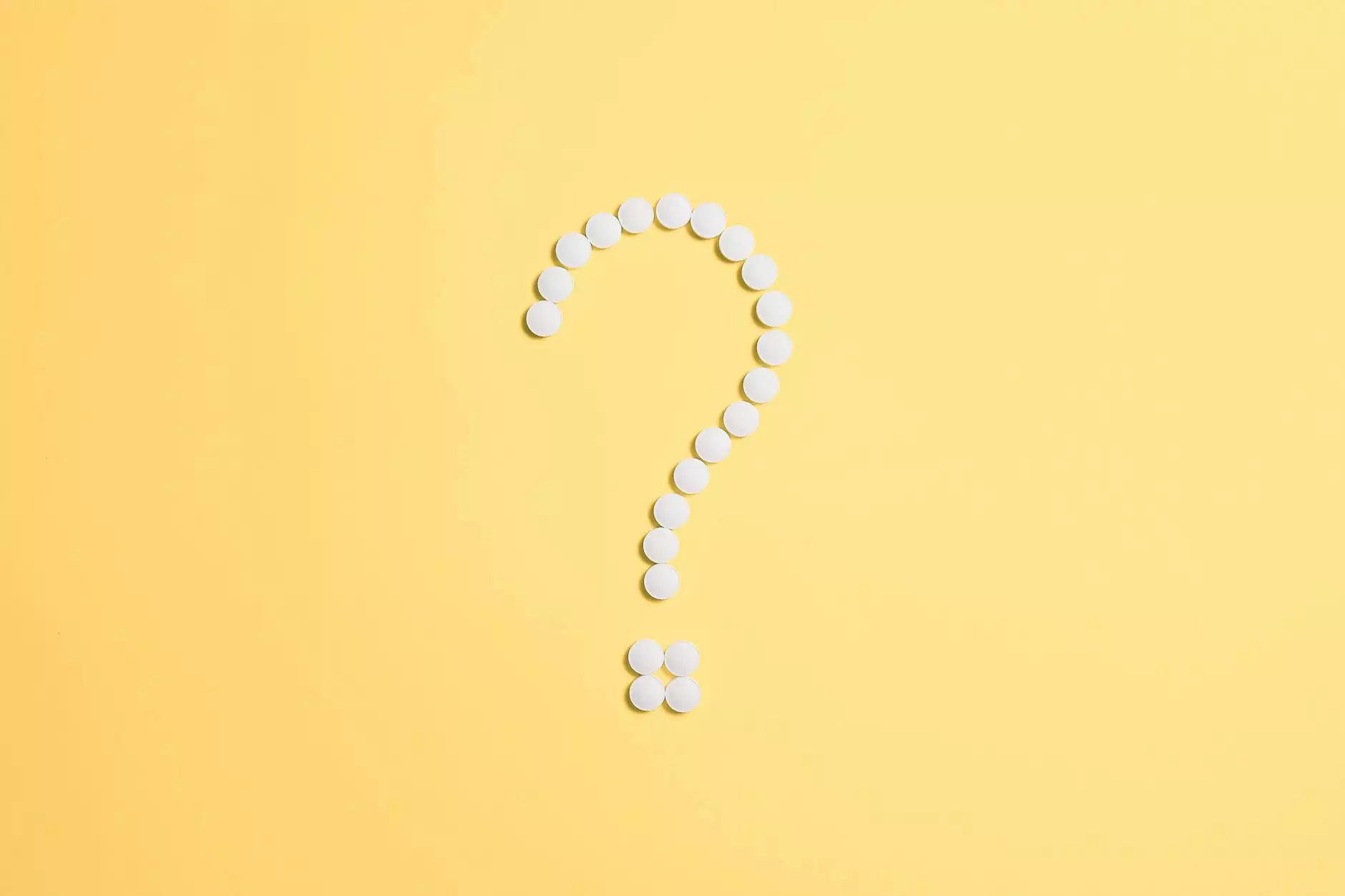
Machine Learning has emerged as a transformative force in various industries, and the field of healthcare is no exception. By leveraging advanced medical datasets for machine learning, professionals are now able to enhance diagnostic accuracy, tailor treatment plans, and improve overall patient care. This article explores the importance of these datasets, their applications, and the positive impacts they have on the medical industry.
Understanding Medical Datasets for Machine Learning
A medical dataset comprises a collection of structured and unstructured data collected from various healthcare sources, including hospitals, clinics, research institutions, and health surveys. These datasets are essential for training machine learning models, enabling computers to recognize patterns, make predictions, and ultimately support clinical decision-making.
Types of Medical Datasets
Medical datasets can vary widely, encompassing numerous types of information. Some of the most prevalent include:
- Electronic Health Records (EHRs): These contain patient history, medications, allergies, lab results, and more.
- Medical Imaging Datasets: Images collected from MRIs, X-rays, CT scans, and ultrasounds used for training models in image recognition.
- Genomic Data: Information derived from genome sequencing which aids in personalized medicine and genetic research.
- Clinical Trial Data: Data collected during clinical trials, which aids in understanding drug efficacy and patient responses.
- Patient Surveys and Questionnaires: Subjective data reflecting patient experiences and outcomes.
The Significance of Quality Data in Machine Learning
The efficacy of machine learning models in the medical field heavily relies on the quality of training datasets. High-quality datasets enable more accurate and reliable predictions, leading to better patient outcomes. Consider the following factors:
- Data Accuracy: Accurate data ensures that the model learns the correct patterns.
- Diversity: A diverse dataset helps in generalizing the model across different patient demographics.
- Completeness: Datasets should cover a complete spectrum of conditions and patient histories.
Applications of Machine Learning in Medicine
The integration of machine learning into healthcare is revolutionizing various aspects, ranging from predictive analytics to personalized medicine. Here’s a closer look at the applications:
1. Predictive Analytics in Patient Care
Machine learning algorithms can predict patient outcomes based on historical data. For example, by analyzing a patient's clinical history and demographic information, models can forecast risks such as readmission or disease progression. This allows healthcare providers to proactively intervene, ultimately leading to improved patient outcomes.
2. Drug Discovery and Development
Utilizing medical datasets for machine learning accelerates the drug discovery process. By examining existing datasets, researchers can identify potential drug candidates, predict their interactions, and determine their efficacy, all while significantly reducing the time and cost associated with traditional drug development methodologies.
3. Personalized Medicine
Machine learning allows for personalized treatment plans based on individual patient data. By analyzing genetic, environmental, and lifestyle factors, healthcare professionals can craft treatment strategies tailored to the unique characteristics of each patient, leading to better therapeutic outcomes.
4. Medical Imaging and Diagnostics
Machine learning algorithms are increasingly being used in medical imaging for tasks such as identifying tumors or other abnormalities in radiological images. These models can assist radiologists in detecting conditions that may be missed by human eyes, thereby improving early diagnosis rates.
5. Operational Efficiency in Healthcare
Institutions can also employ machine learning to enhance operational efficiency. For instance, predictive analytics can forecast patient admission rates, enabling hospitals to allocate resources more effectively and maintain a high level of patient care.
Challenges in Utilizing Medical Datasets
While the advantages of machine learning in the medical field are clear, certain challenges must be addressed:
- Data Privacy: Protecting patient information is paramount, and healthcare providers must ensure compliance with regulations such as HIPAA.
- Bias in Datasets: If datasets are biased, the models trained on them may produce skewed results, potentially leading to unequal treatment across different patient populations.
- Integration with Existing Systems: Many healthcare facilities face difficulties in integrating machine learning technologies into their established workflows.
The Future of Machine Learning in Healthcare
The future prospects for machine learning in healthcare are indeed promising. Innovations such as real-time data analytics, non-invasive diagnostic techniques, and remote patient monitoring are on the horizon. As healthcare continues to evolve through technological advancements, the role of machine learning is expected to expand, facilitating a paradigm shift toward predictive, personalized, and preventive care.
Conclusion
In conclusion, the use of medical datasets for machine learning is a game-changer in the healthcare field. By harnessing the power of these datasets, we can improve patient care, enhance clinical research, and foster innovation within the industry. As we move forward, it is essential to address the challenges inherent in data utilization and ensure that advancements in machine learning are accessible to all, ultimately leading to a healthier future for everyone.
As we continue to make strides in this exciting domain, it's crucial for businesses in the healthcare sector, including those involved in Home Services, Keys & Locksmiths like keymakr.com, to stay informed about these developments and consider how they can integrate machine learning into their services. The journey of innovation in healthcare is just beginning, and the potential is limitless.
medical dataset for machine learning